In the first and second post about my Bayesian model to rank NBA referees I committed a grave statistical sin: I reported parameter estimates without indicating the amount of uncertainty involved. Although I alluded to the confidence intervals being large, I selfishly didn't want to emphasize them in my first two posts because I felt like they were a story in their own right and would detract from the story I was trying to tell about the overall model building process. So in this post I repent and make things right by looking at (1) the parameter estimate uncertainty, (2) the uncertainty in the ranks among referees (and why Bayesian stats makes that so easy!), and (3) the hypothetical uncertainty we would have if the NBA drastically expanded their Last Two Minute reports.
Parameter Estimate Uncertainty
The great thing about a fully specified Bayesian model (estimated using MCMC) is that uncertainty estimates and confidence intervals come easily as a by-product. Our MCMC chains represent a sample from our posterior distribution, so we can use that sample to calculate just about any quantity of interest for our posterior. The most natural quantity for our model is a 95% credible interval on the rate estimated for each referee in our dataset. Here I show equal-tailed credible intervals:
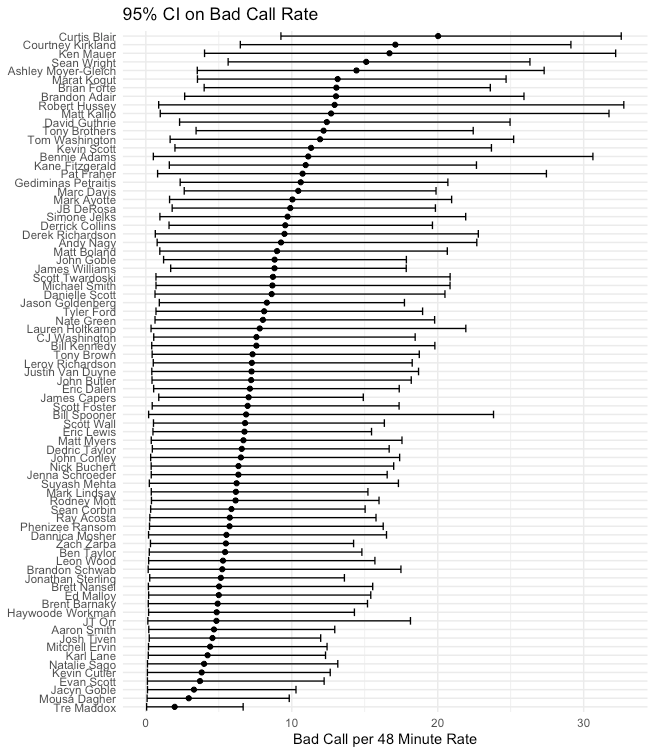
Usually it's a bad sign when your confidence intervals take over most of the axis of the plot. Unfortunately what the model is telling us is that almost every referee in the league could plausibly be making about ten bad calls a game. Or not. Maybe some referees are making 1-2 bad calls a game, and the others are making 30. The purpose of checking the confidence intervals are to be able to know what we don't know, and there's a lot we don't know given the small dataset we have. I have two thoughts on why these confidence intervals are so large:
The dataset is only 400 games, mostly consisting of only two minutes of those 48 minute games (although occasionally more than 2 minutes due to overtime). This is about 1/3rd of the total games played in the NBA, and 1/24th of the time. Roughly speaking, the L2M reports cover a paltry 1.5% of all game time in the NBA. And we have to use that 1.5% to judge the performance of 79 referees. Not an easy task.
A large part of the uncertainty comes from who is to blame for each bad call. Each game has three referees on the court. The parameters estimated in the model attempt to estimate the unique contribution of each ref, but that's highly obfuscated by the other refs who might also be to blame for the bad calls while that ref was on the court. The model is capturing the attribution uncertainty and reflecting that in its estimates.
Rank Uncertainty
We usually think of confidence intervals on the parameters in our model, but it's a lot more useful to be able to convert those confidence intervals to our actual questions of interest. This model was not built with the intention to understand how many bad calls were being made, instead it was made to provide an actual ranking to each of the 79 referees. Additionally, just because two rate credible intervals overlap does not necessarily mean there is high uncertainty in which referee is better. If the correlation was extremely high between the two refs, the credible intervals on the rate parameter might overlap substantially while the probability that Ref A is better than Ref B is extremely low. This doesn't seem to be the case here, but it came up frequently when I analyzed MaxDiffs at Qualtrics.
The rank is a mathematical transformation on the rate parameters we estimated in our model, so we can use the posterior samples to calculate the credible intervals after applying this transformation. If we were working with a frequentist model we could probably use a bootstrap approximation, but this is much easier using a Bayesian framework where we can sample our posterior:
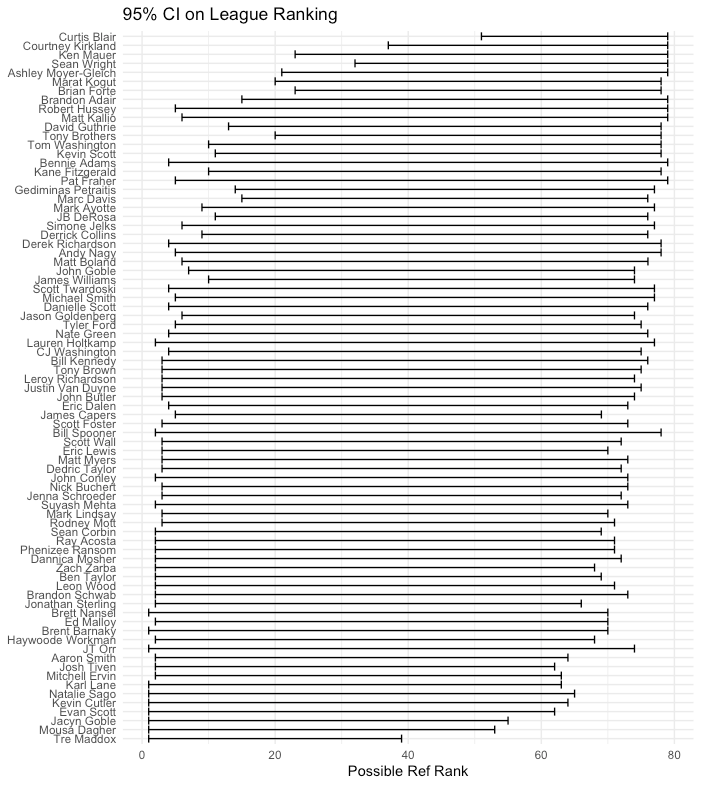
While the first graph was concerning this one is downright disheartening. While I can confidently say I'd rather have Tre Maddox referee my game than Curtis Blair, almost any other conclusions are still mired in uncertainty. We just don't have the data to provide definitive statements about which referees are performing well in the NBA.
Hypothetical Uncertainty
This model allows us to ask the question: what would the NBA need to do to provide enough transparency to accurately rank the referees in the league? As an experiment, I simulated the uncertainty we would have if we kept the same number of games in our dataset (400), but expanded time under review to the full 48 minutes instead of the last 2 minutes. For the simulation I just multiplied the number of bad calls in the last two minutes by 24 to simulate how many bad calls might be identified in the full 48 minutes. The new uncertainty plot looks like:

Now that's much better. While we still aren't able to provide an exact ranking for every referee in the league, we can confidently assign a tier to each of the referees. If the NBA assessed referees this thoroughly, it could easily make rules about where your rank has to be to ref a playoff or finals game. Additionally, in this scenario it is much easier to see which referees hadn't been assessed adequately, like Bill Spooner who has a huge range because he only reffed one game in our dataset.
Conclusion
I see a lot of sports analysts on Twitter that create all sorts of rankings of players for all sorts of statistics. What's typically missing from these rankings is any indication of how significant the differences between the players actually are. Confidence intervals help us distinguish what we might experience from random variation vs. real signal in the data, and they're a meaningful first step in getting more actionable insights from sports analytics.
As they say in the NBA, "Tre Maddox: Probably a good ref, but maybe not!™"