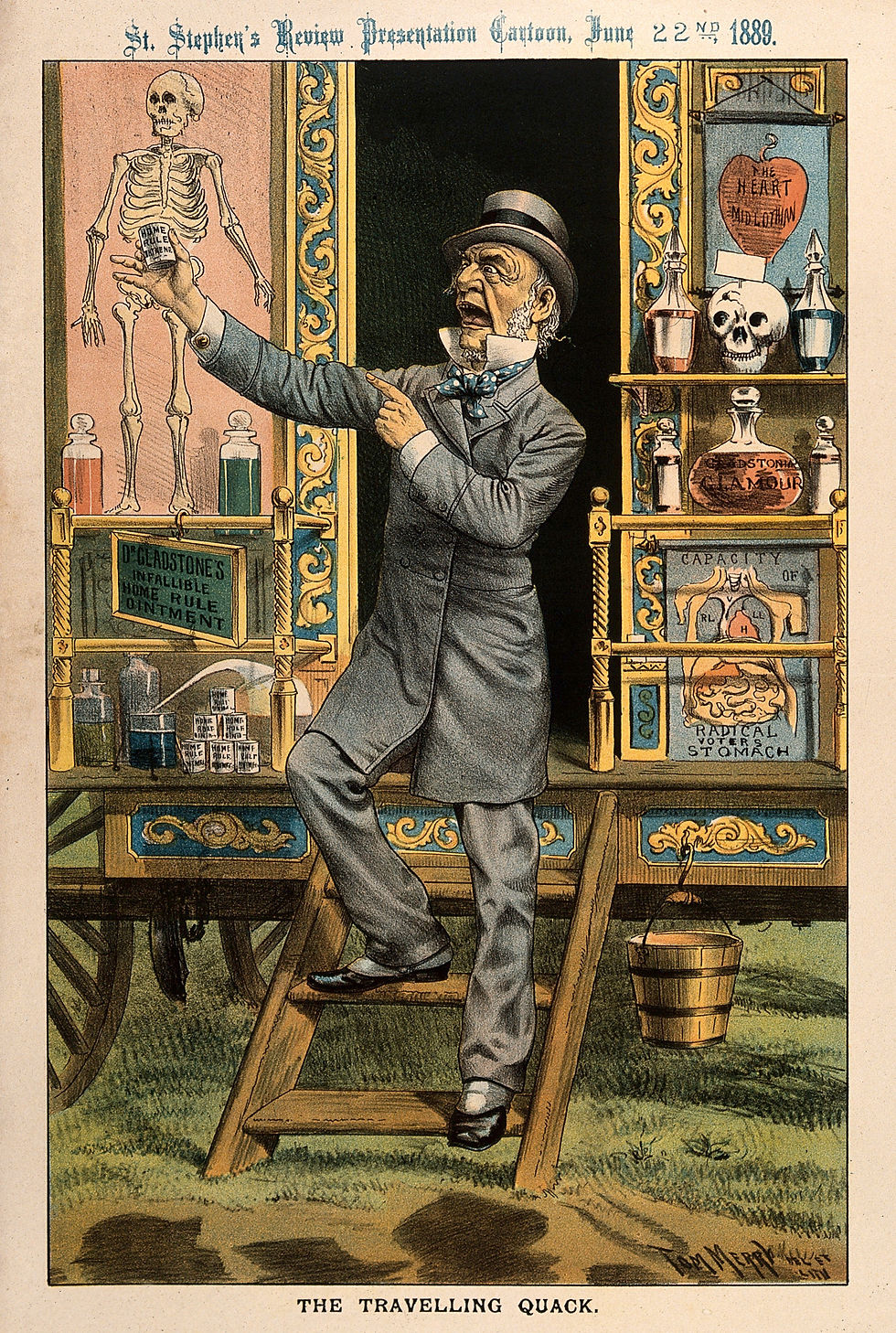
My tooth was hurting the other day, and I was trying to figure out how to take care of it without going to the dentist. I don't have anything against dentists except that they aren't covered by medical insurance. I imagined talking to my doctor about my tooth problem (in hopes of getting a solution I could bill to insurance), but realized that was pretty silly since he'd instantly refer me to a dentist. However, I think there are doctors (and tons of people online) who would be more than happy to sell me a tooth pain remedy: quack doctors.
One thing that characterizes a medical quack is a general belief that a small set of solutions can solve a nearly infinite set of medical issues. Have a migraine? Use this essential oil. Want to stay safe from COVID? Three drops of this each morning will do the trick. Fertility issues? You get the idea. In contrast, a good general medicine doctor knows exactly when it's time to send her patients to a specialist. They recognize that the medical field is way too wide for them to have detailed knowledge necessary to solve every issue that might come up in health. Doctors embrace specialization and have a (mostly) effective solution for routing to the person most likely to be able to help the patient.
One of the most common complaints I see in data science, from both the business side and the data scientists themselves, is that they aren't having a meaningful impact on the business. I think a lot of this failure to produce real results is because data scientists act too much like the quacks and not enough like the doctors. Too often, data scientists see themselves as holder of special knowledge others don't have access to, they assume their tools are perfect for solving any problem, and they reject specialization out of a belief that all non-data science tools are inferior to what they have. While we all adopt some of these attitudes some of the time, I think the following points can be used as a litmus test to measure where you fall on the spectrum of doctor vs. quack:
Selling a placebo - Data science is a huge field encompassing concepts from math, statistics, computer science, and more. We would never want our doctor to assume he could "figure out" brain surgery once a patient needs it, yet we seem to embrace the idea that a data scientist can read a blog post or journal article and we will be completely competent in it the first time.
Over-reliance on a single "cure-all" (the predictive model) - Like those essential oil folks who have a different flavor oil for every malady, data scientists seem to assume that every data problem can be solved by some flavor of a predictive model. I can't tell you how many times I've had to have this fight: No amount of explainable AI will turn a predictive model into a causal one automatically. Data scientists who can't clearly explain why effective decisions follow logically from their analysis aren't going to earn trust in an organization.
Us vs them attitude - Alternative medicine sustains itself on the belief that regular medicine is evil or has failed. Too often I see data scientists take the same view of their own business partners because they don't solve problems in the same way we do. I've found the safest assumption is to assume that everyone in the business is making nearly optimal decisions through sheer trial and error and then working on incremental improvements.
Lack of clarity around offerings - A good doctor will always walk you through what's going to happen to you before you go into surgery. This builds trust and confidence. Too often, data scientists don't have a well-defined plan for what they offer and what benefits it brings. We expect people to bring us problems and trust our solutions that they can't understand. Business people are going to come to you when they are confident they have a problem you solve for, so when you lack this clear messaging about your procedures, it is much more likely you'll be remembered only as "the people who helped me track down this number to go in my Powerpoint."
Rejecting other necessary skillsets - In data science, I've seen a general lack of willingness to build the skillset to specialize on specific problem areas in a business. "In the end, it's all just numbers" is the typical reply from data scientist. But if you don't truly understand the problems in the subject areas you're working in (demand planning, sales ops, etc), you'll always be relying on others to tell you the problems that need solved and the relevant data.
I think a reasonable debate can be had about many of the points above. For example, we probably don't want data scientists to have eight years of training in a specific methodology before applying their skills like we do for doctors. However, with the number of data scientists I know who are unhappy about the impact their efforts have on the company, more carefully thought out methodology and more deeply embedding data scientists in their problem areas could go a long way to increasing their effectiveness.
What do you think? Is there anything you'd add to this list? Anything you'd remove? Share your experiences with me:
If you work in data science, what problems do the the rest of the business come to you for? What problems do they think you solve? Is that list what you want it to be?
Should data science be more applied? Should data science be centralized with a consultant model? Or should they be embedded on teams throughout the organization?
Should data science have more or less hesitancy in pitching methodologies they've never used? Is it okay to turn down projects you don't think will produce value?
Can a data scientist working in a quack culture change it?
What project that you worked on provided the single biggest ROI to the business?
Comentarios